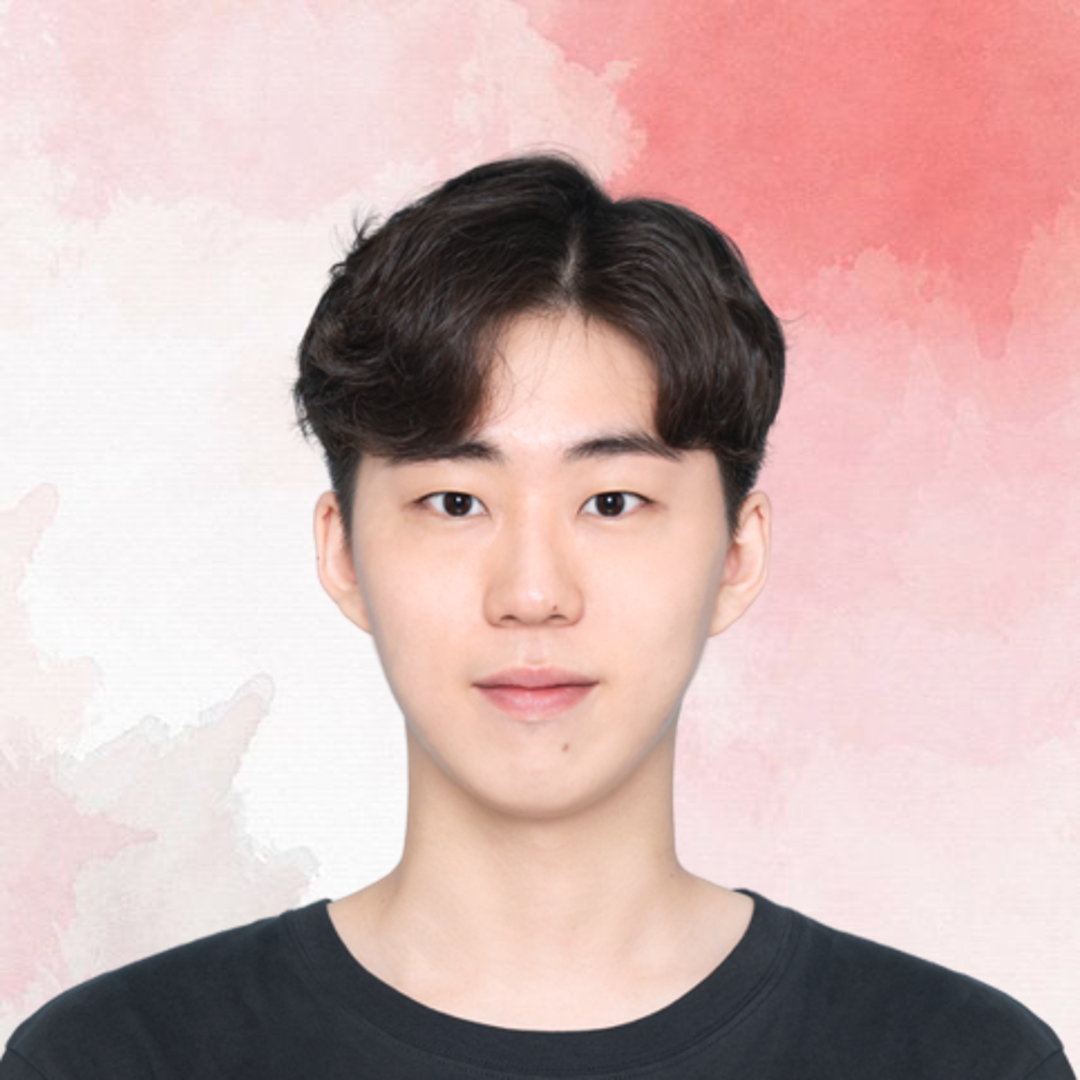
OptiML Lab / KAIST AI
Jaewook Lee
Biography.
Hello, my name is Jaewook Lee. (In English I go by David!) I am a Master's Student in the Optimization and Machine Learning (OptiML) Laboratory, fortunate to be advised by Prof. Chulhee Yun at KAIST AI. Beforehand, I completed my B.S. in Electrical Engineering and Mathematical Sciences (Double Major) at KAIST.
I am interested in optimization theory including both convex/nonconvex & stochastic optimization algorithms and applications to practical settings in AI and/or deep learning theory. Recently I have been particularly interested in Wasserstein gradient flows. I am also interested in minimax optimization and similar topics like control/operator theory & variational inequalities, multi-player games & multi-agent learning, and block coordinate descent (which could be thought of as a purely cooperative n-player game). I am also always eager to learn more about any other interesting optimization, ML/DL theory or math-related topics!
Education.
-
Current
Korea Advanced Institute of Science and Technology, Seoul, South Korea
M.S. in Artificial Intelligence
GPA: 4.25/4.3
-
Feb 2023
Korea Advanced Institute of Science and Technology, Daejeon, South Korea
B.S. in Electrical Engineering & Mathematical Sciences (Double Major)
GPA: 4.07/4.3, Summa Cum Laude
Graduated with Excellence in Leadership & Volunteering
-
Feb 2018
Graduated Sejong Science High School, Seoul, South Korea
Topics of Interest.
Convex/Nonconvex Optimization
I am interested in theoretical analysis and design of deterministic/stochastic optimization algorithms for convex and nonconvex functions with faster convergence and/or computational efficiency.
Minimax Optimization
I am interested in minimax optimization algorithms, similar problem classes including fixed point problems or variational inequalities, and broader related topics including multi-player games and multi-agent learning.
Wasserstein GF
I am interest in optimal transport theory and Wasserstein gradient flows. In particular, I am studying optimization algorithms on Wasserstein spaces and applications to deep learning theory as in mean field neural networks.
Optmization for ML/DL
I am interested in applying optimization & theoretical perspectives to machine/deep learning problems, including theoretical analysis and the optimization dynamics of transformers or diffusion models.
Publications.
-
Fundamental Benefit of Alternating Updates in Minimax Optimization
Jaewook Lee*, Hanseul Cho*, Chulhee Yun
International Conference on Machine Learning (ICML) 2024
Spotlight Paper, Top (144+191)/9473=3.54% of papers
-
Tighter Lower Bounds for Shuffling SGD: Random Permutations and Beyond
Jaeyoung Cha, Jaewook Lee, Chulhee Yun
International Conference on Machine Learning (ICML) 2023
Oral Presentation, Top 155/6538=2.37% of papers
-
*Equal Contribution
Experience.
My main research topic in OptiML Lab was the investigation of worst-case convergence lower bounds of gradient-based optimization algorithms, which involves convergence analysis in pathological cases specifically designed for the algorithm to show its worst performance.
In MLILAB, I mainly studied and implemented visual data generation models based on 3D morphable face models and neural renderers, specifically aiming to achieve better-performing expression/identity swapping between different images or frames, such as talking head generation or face swapping.
Awards & Honors.
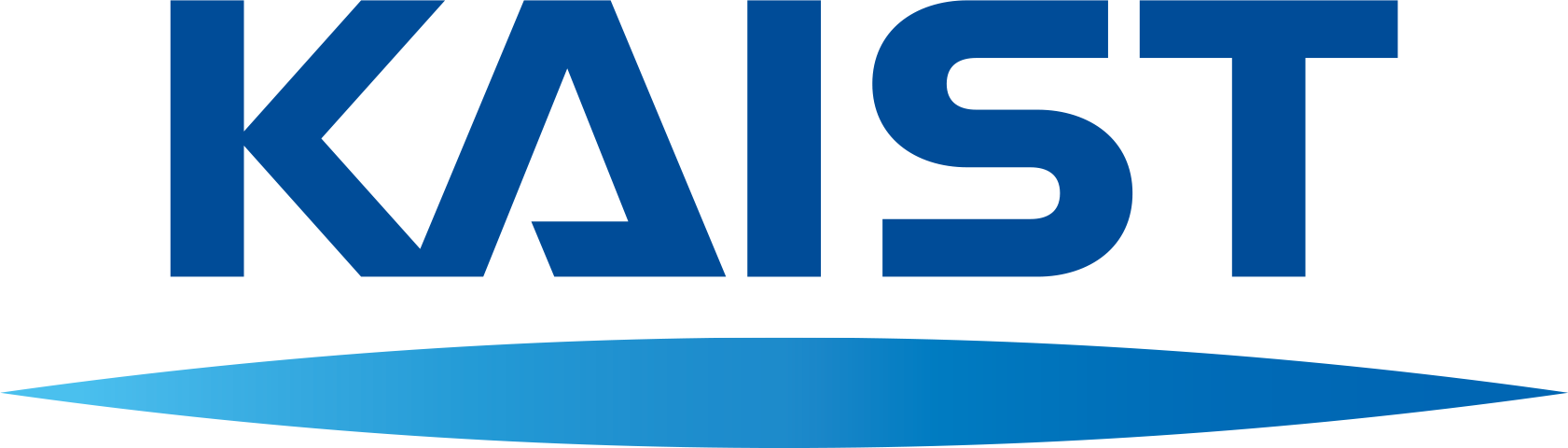
KAIST Math PoW: 3rd Prize - Fall 2021
Weekly math competition in KAIST, open to all undergraduate/graduate students
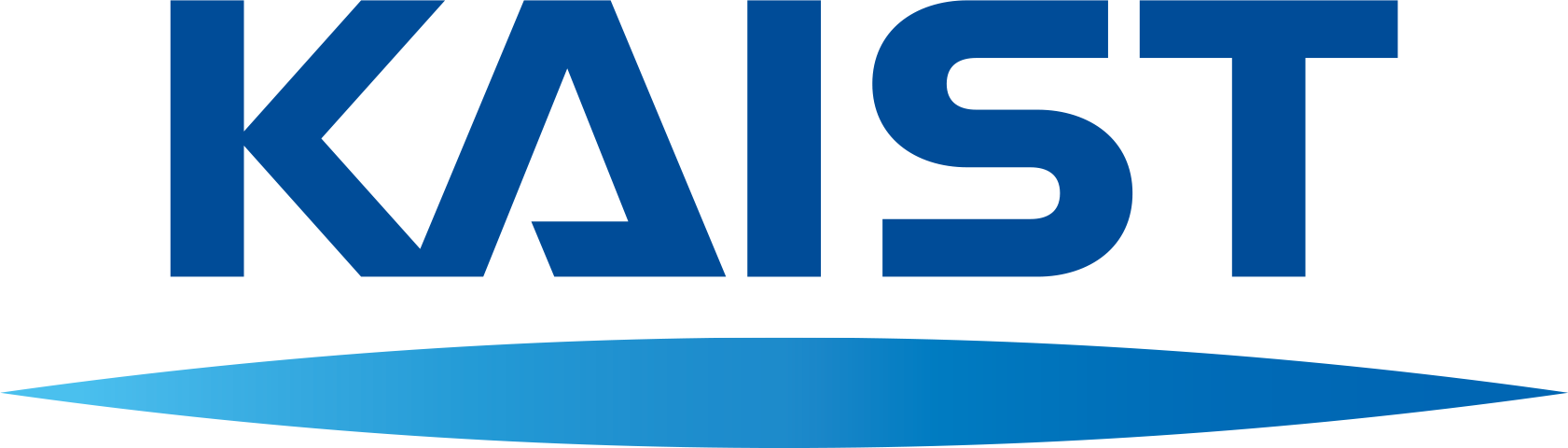
Academic Excellence Scholarship, KAIST - Fall 2020
Scholarship, awarded to the top 4 students in KAIST EE
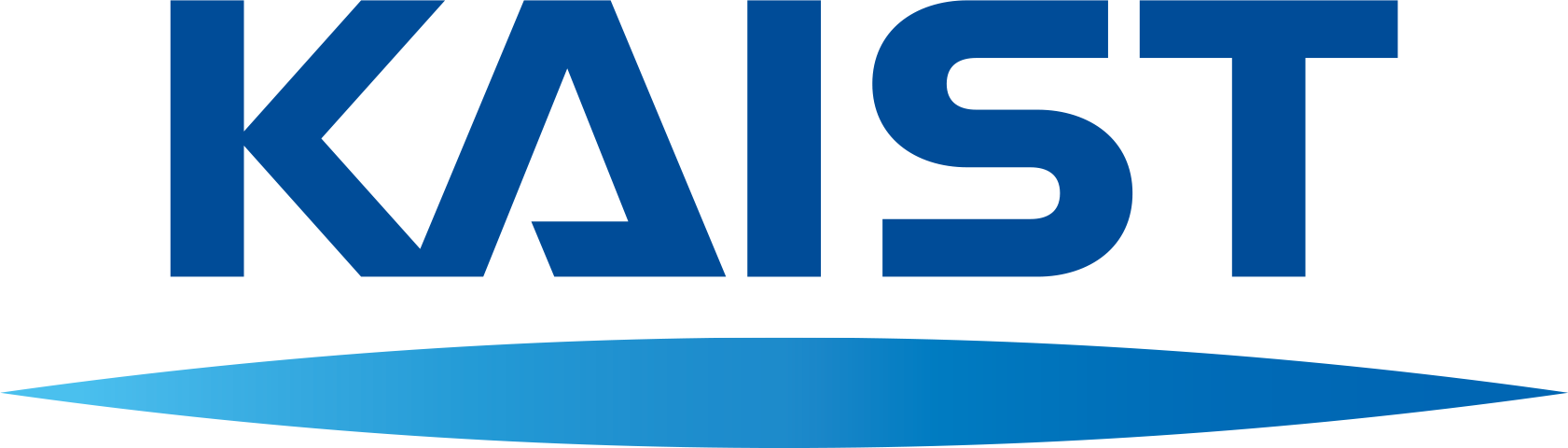
Dean's List Award, KAIST - Fall 2019, Fall 2020, Spring 2021
Awarded to the top 2% students in KAIST EE
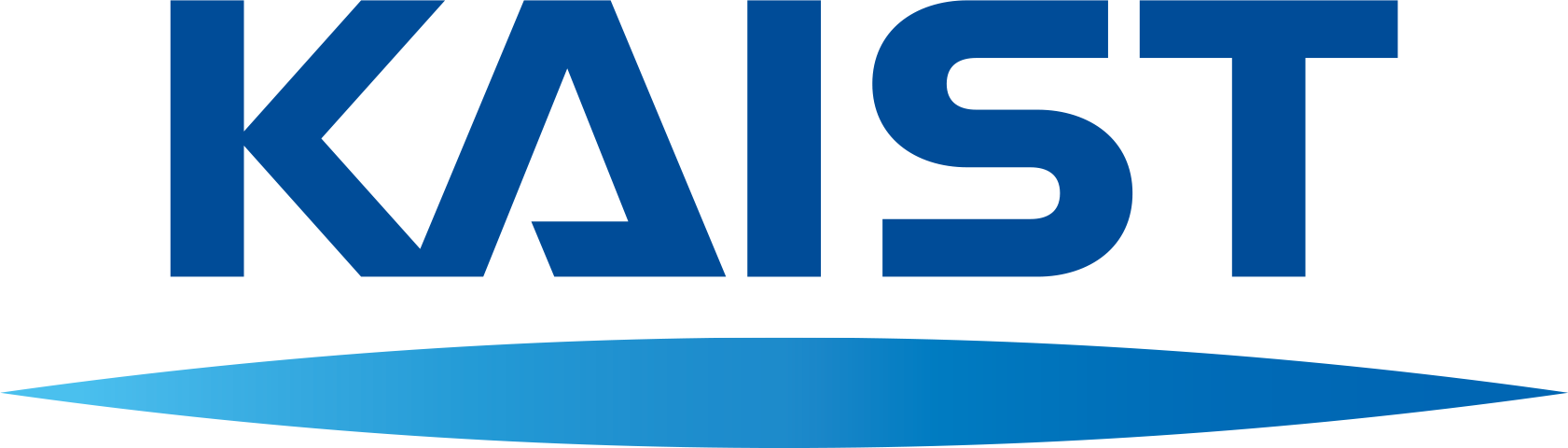
Freshman Dean's List Award, KAIST - Fall 2018
Awarded to the top 2% students among KAIST freshmen
Contact.
- 99rma37@kaist.ac.kr
- +82-10-3539-1857
- 85 Hoegi-ro, Dongdaemun-gu, Seoul, South Korea